On October 25, 2024, Professor Yin Ping's team from the School of Public Health, Tongji Medical College, Huazhong University of Science and Technology, published a methodological paper titled "A Modified Debiased Inverse-Variance Weighted Estimator in Two-Sample Summary-Data Mendelian Randomization" in the prestigious journal Statistics in Medicine (https://doi.org/10.1002/sim.10245). This method, grounded in bias-correction theory, constructs a novel two-sample Mendelian randomization (MR) approach by multiplying the dIVW (debiased inverse-variance weighted) estimator with a shrinkage factor. Compared to the dIVW method, the new approach achieves smaller bias and variance and is more robust in scenarios with extremely weak instrumental variables (IVs).
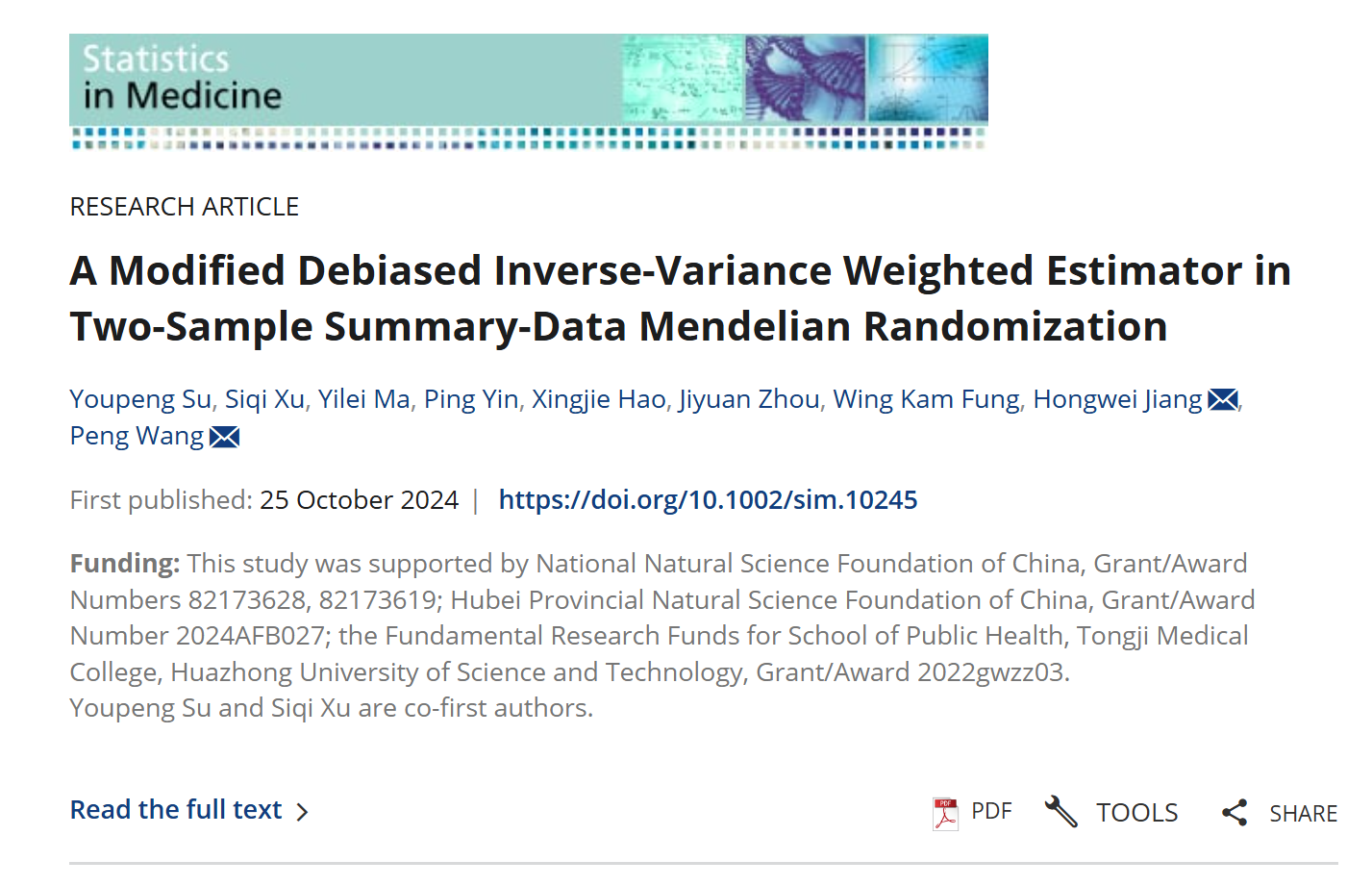
MR uses genetic variants as IVs to estimate the causal effect of exposure on outcome from observational data. Unlike traditional IV methods, a distinct feature of MR is that there can be a large number of genetic variants from preexisting large genome-wide association studies (GWASs), and many or possibly all SNPs are weak IVs; the setting is also referred to as many weak instruments in econometrics. Many traditional MR methods, such as the IVW method, may introduce weak instrument bias in this scenario.
Accordingly, based on the IVW estimator, two recently proposed methods, the debiased IVW (dIVW) estimator and the penalized IVW (pIVW) estimator demonstrate robustness to weak IVs. However, we find that the dIVW estimator tends to overestimate causal effects, while the pIVW estimator is more complex and lacks intuitive interpretation. To address these issues, our team propose a modified dIVW (mdIVW) estimator by applying a shrinkage factor to the dIVW estimator. After this simple modification, we show that the mdIVW estimator has the smallest variance, and its bias is only of second order with respect to the effective sample size. Although both the mdIVW and pIVW estimators have the same order of bias, the former has a simpler form and clearer rationale. Additionally, the second-order relative bias of mdIVW estimator is always negative, which can help avoid false positive results.
Through theoretical derivation, numerical simulations, and real data analysis, we demonstrate that the new method offers advantages in terms of bias and variance, thereby providing a more robust and efficient option for two-sample MR studies.
Journal Introduction
Statistics in Medicine journal enhances communication between statisticians, clinicians and medical researchers, influencing and improving practice in medicine and its associated sciences. It is also recognized as a T2 journal in probability and statistics by the Chinese Mathematical Society High-Quality Science and Technology Journal Ranking (2024).
Author Information
Youpeng Su, a PhD candidate of the School of Public Health, is the first author of the study. Lecture Peng Wang, PhD and Associate Professor Hongwei Jiang are co-corresponding authors. This research was supported by the National Natural Science Foundation and the Fundamental Research Funds for School of Public Health, Tongji Medical College, Huazhong University of Science and Technology.